|
|
Judea Pearl's new book, The Book of
Why, is a must read for anyone interested in philosophy, science,
machine learning or statistics. [Milo Schield, Editor]
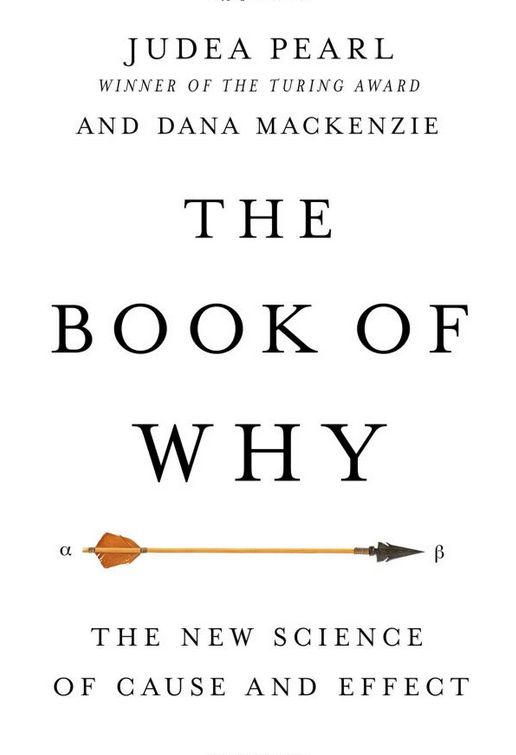
Judea Pearl website
List of papers.
The Book of Why: The New Science of Cause and Effect.
Summary and TOC.
Index
Pearl's update.
Causality (2nd ed) website:
1. Why I wrote this book 2. Table
of Contents 3. Preface 4. Preview of text Important Topic: On the meaning of
structural equations (from Causality, Sections 5.3.2 - 5.4.1) 5. Reviews
6. Discussion with readers 7. Viewgraphs and homework for instructors 8.
Recent seminars 8.1 video from a UCLA seminar on the state of causality in
economics 8.2 video from a seminar given at John Hopkins University 8.3
video from a seminar given at ISI 8.4 video of lecture on causes and
counterfactuals 9. Lakatos Award for 2001 10. Excerpts from the 2nd edition
of Causality
An Introduction to Causal Inference by Judea Pearl.
February 8, 2015
The Art and
Science of Cause and Effect
Transcript and slides of 1996 Faculty Research Lecture.
Reasoning with Cause and Effect
Transcript and slides of 1999 IJCAI
Award Lecture.
Understanding Simpson’s Paradox
(2014)
The American Statistician, February
2014, Vol. 68, No. 1.
Simpson’s paradox
is often presented as a compelling demonstration of why we need statistics
education in our schools. It is a reminder of how easy it is to fall into a
web of paradoxical conclusions when relying solely on intuition, unaided by
rigorous statistical methods. In recent years, ironically, the paradox
assumed an added dimension when educators began using it to demonstrate the
limits of statistical methods, and why causal, rather than statistical
considerations are necessary to avoid those paradoxical conclusions
(Wasserman 2004; Arah 2008; Pearl 2009, pp. 173–182).
My comments are
divided into three parts. First, I will give a brief summary of the history
of Simpson’s paradox and how it has been treated in the statistical
literature in the past century. Next, I will ask what is required to declare
the paradox “resolved,” and argue that modern understanding of causal
inference has met those requirements. Finally, I will answer specific
questions raised in Armistead’s article and show how the resolution of
Simpson’s paradox can be taught for fun and progress.
The Causal
Mediation Formula – A Guide to the Assessment of Pathways and Mechanisms
(2012)
Abstract: "Recent advances in causal inference
have given rise to a general and easy-to-use formula for assessing the
extent to which the effect of one variable on another is mediated by a
third. This Mediation Formula is applicable to nonlinear models with both
discrete and continuous variables, and permits the evaluation of
path-specific effects with minimal assumptions regarding the data-generating
process. We demonstrate the use of the Mediation Formula in simple examples
and illustrate why parametric methods of analysis yield distorted results,
even when parameters are known precisely. We stress the importance of
distinguishing between the necessary and sufficient interpretations of
“mediated-effect” and show how to estimate the two components in nonlinear
systems with continuous and categorical variables."
Keywords and phrases: Effect decomposition,
direct and indirect effects, structural equation models, percentage
explained, moderation
Contents: 1) Introduction 2)
Total, direct and indirect effects 3) The Mediation Formula: A
Simple Solution to a Thorny Problem 4) Relations to
Traditional Approaches 5) Conclusions
Excerpts: "Consider a randomized clinical
trial in which an intervention X shows a significant effect on an outcome Y
. A question that invariably comes to investigators’ minds is: How and why
does the intervention produce the effect, or, more specifically, can the
effect of X on Y be attributed to its effect on some intermediate variable Z
standing between the two?"
A. M.
Turing Award Winner: 2011 "For
fundamental contributions to artificial intelligence through the development
of a calculus for probabilistic and causal reasoning."
Video.
Statistics and Causality: Separated to Reunite. Commentary on
Bryan Dowd's 'Separated at Birth' (2011)
Excerpt: "I see the tension between statistics and
economics or, more generally, between statistics and causality, to be rooted
in a more fundamental schism than the one portrayed in Dowd’s account.
Moreover, and contrary to Dowd’s narrative, I believe that the schism was
justified, necessary, and not sufficiently emphasized. In fact, it was only
after the distinction between statistical and causal concepts was made crisp
and formal through new mathematical notation that a productive symbiosis has
emerged which now benefits both paradigms."
Conclusion: "Causal and
statistical information are two different species that do not and should not
be mixed. The latter deals with probabilistic relationships among observed
variables; the former deals with hypothetical relationships in new
situations. These relationships should be kept apart by notational
distinctions and be governed by separate calculi. Once the mathematical
distinction is accomplished, symbiotic analysis can benefit both causal and
statistical inferences."
Causal Inference
in Statistics: An overview (2009)
Abstract: This review presents empirical researchers with
recent advances in causal inference, and stresses the paradigmatic shifts
that must be undertaken in moving from traditional statistical analysis to
causal analysis of multivariate data. Special emphasis is placed on the
assumptions that underly all causal inferences, the languages used in
formulating those assumptions, the conditional nature of all causal and
counterfactual claims, and the methods that have been developed for the
assessment of such claims. These advances are illustrated using a general
theory of causation based on the Structural Causal Model (SCM) described in
Pearl (2000a), which subsumes and unifies other approaches to causation,
and provides a coherent mathematical foundation for the analysis of causes
and counterfactuals. In particular, the paper surveys the development of
mathematical tools for inferring (from a combination of data and
assumptions) answers to three types of causal queries: (1) queries about the
effects of potential interventions, (also called “causal effects” or “policy
evaluation” ) (2) queries about probabilities of counterfactuals, (including
assessment of “regret,” “attribution” or “causes of effects”) and (3)
queries about direct and indirect effects (also known as “mediation”).
Finally, the paper defines the formal and conceptual relationships between
the structural and potential-outcome frameworks and presents tools for a
symbiotic analysis that uses the strong features of both
Keywords and phrases: Structural equation
models, confounding, graphical methods, counterfactuals, causal effects,
potential- outcome, mediation, policy evaluation, causes of effects
Contents: 1) Introduction 2)
From association to causation 3) Structural models, diagrams,
causal effects, and counterfactuals 4) The potential
outcome framework 5) Counterfactuals at work 6)
Conclusions
Excerpts: "The questions that motivate
most studies in the health, social and behavioral sciences are not
associational but causal in nature." "although much of the conceptual
framework and algorithmic tools needed for tackling such problems are now
well established, they are hardly known to researchers who could put them
into practical use. The main reason is educational."
"A useful demarcation line that makes the
distinction between associational and causal concepts crisp and easy to
apply, can be formulated as follows. An associational concept is any
relationship that can be defined in terms of a joint distribution of
observed variables, and a causal concept is any relationship that
cannot be defined from the distribution alone. Examples of associational
concepts are: correlation, regression, dependence, conditional independence,
likelihood, collapsibility, propensity score, risk ratio, odds ratio,
marginalization, conditionalization, “controlling for,” and so on. Examples
of causal concepts are: randomization, influence, effect, confounding,
“holding constant,” disturbance, spurious correlation,
faithfulness/stability, instrumental variables, intervention, explanation,
attribution, and so on. The former can, while the latter cannot be defined
in term of distribution functions."
Simpson's Paradox: An
Anatomy (1999)
This report discusses the
reversal effect known as Simpson's paradox from a causal-theoretic
viewpoint. It analyzes the reasons why the effect has been (and still
is) considered paradoxical and why its resolution has been so late in
coming. The report is extracted from a forthcoming book, Causality
[Pearl, 2000] and assumes some familiarity with causal diagrams and the dot
(.) (or set (.))
notation (e.g., [Pearl 1995])
|